Trust and responsibility in AI systems has emerged as both a competitive imperative and social necessity. Practical pathways are required to traverse opaque technology and complex ethical dilemmas. ES/Xcelerate Data TRiSM Framework – The Level 1 presents an accessible entry point – a common language and logic model for business leaders to lead inclusive advancement of AI in their organization.
Grounded in global standards and policy, the Framework integrates complementary guidance into a consolidated resource for executive education, strategy, and governance. The modules empower leadership to set the tone, allocate resources to priorities, and coordinate specialized teams accountable for ethical AI delivery. The focus elevates beyond principles into pragmatism, backed by continuous benchmarking.
This people-centric structure fosters achievable adoption tailored to risk profiles and use cases without over-indexing strict mandates. Progress follows principles-based guardrails, not prescriptiveness. Success necessitates cultural commitment, not just conceptual compliance. Shared fundamentals orient varied voices to drive transparency, explicability, and inclusion throughout AI model development, deployment, monitoring and maintenance. Now is the time for cross-sectional collaboration with compassion. We invite you to join us on the journey.
License
The Level 1 Framework guidance is published under a Creative Commons Attribution 4.0 International license (CC BY 4.0). This allows the ability for others to share or adapt the material for any purpose, even commercially, as long as appropriate credit is given. Our aim is to maximize access, sharing, and transparency of the executive education resources to accelerate awareness and adoption of ethical AI practices across industries.
Attribution fuels continued improvement – we welcome feedback from organizations implementing Level 1 recommendations into their awareness training, strategy development, governance models and policy initiatives as we continuously update the framework together with the community. Please reach out as you put these resources to use so we can track impact and needs to inform ongoing enhancement of trustworthy and responsible AI systems.
Introduction to ES/Xcelerate Data TRiSM Framework
We have developed a new TRiSM framework with leading experts that provides modular guidance across executive, technical, and control levels for building reliable, safe, and ethical AI systems. It allows flexible implementation of solid principles through benchmarking, role-based recommendations, and predefined controls suited to an organization’s priorities and context. This adaptable integration enables customized advancement in responsible AI without over-prescription.
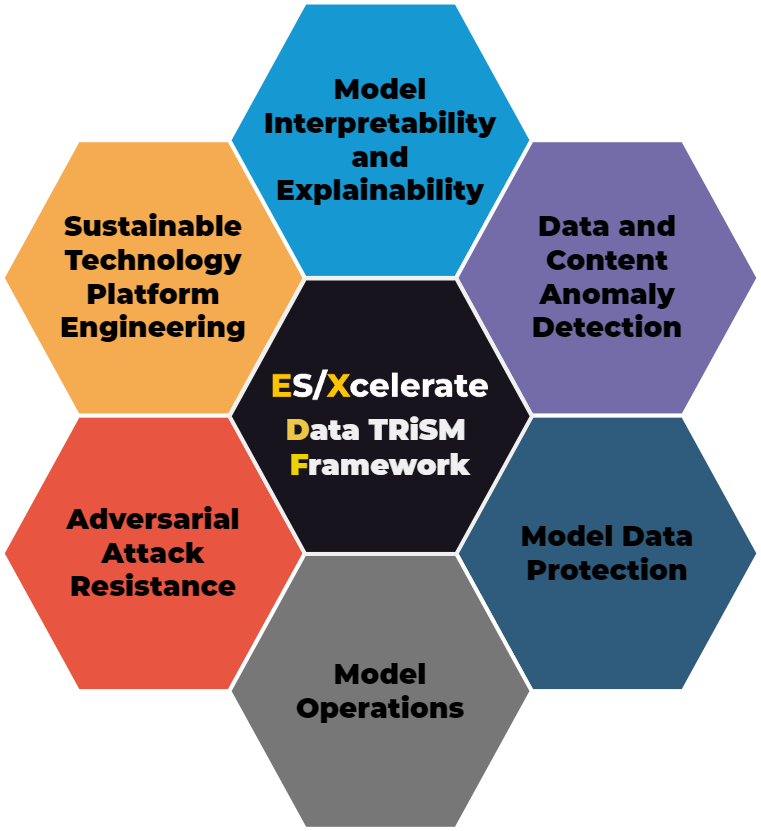
- Model Interpretability and Explainability
- Data and Content Anomaly Detection
- AI Data Protection
- Model Operations
- Adversarial Attack Resistance
- Sustainable Technology Platform Engineering
Model Interpretability and Explainability
Provides transparency into AI systems through explanations of model behavior, features, and decisions to establish trust and ensure human oversight.
Control | Principle | Description |
---|---|---|
1.1 | Explanation | The model should provide clear and understandable reasons for its predictions, decisions and actions, in a way that is suitable for the intended audience. |
1.2 | Meaningfulness | The model should use concepts, terms and features that are relevant and meaningful for the problem domain and the data context. |
1.3 | Accuracy | The model should provide explanations that are consistent, faithful and accurate to the underlying model logic and data. |
1.4 | Knowledge Limits | The model should acknowledge and communicate the limitations, uncertainties and assumptions of its explanations, and avoid overconfidence and overgeneralization. |
1.5 | Sustainability | The model should be designed and operated in a way that minimizes the environmental impact and carbon footprint of data-intensive workloads, while also optimizing the performance and cost efficiency of such workloads. |
Data and Content Anomaly Detection
Enables continuous validation of data and model outputs to identify errors, biases, outliers and deviations from expected patterns.
Control | Principle | Description |
---|---|---|
2.1 | Sensitivity | The method should be able to detect anomalies of different types, scales and patterns, and adjust the detection threshold according to the data characteristics and the application context. |
2.2 | Efficiency | The method should be able to handle large and complex data sets, and provide timely and scalable detection results, while minimizing the computational and storage costs. |
2.3 | Robustness | The method should be able to cope with noise, uncertainty and incompleteness in the data, and avoid false positives and false negatives in the detection outcomes. |
2.4 | Interpretability | The method should be able to provide clear and understandable explanations for the detected anomalies, and highlight the relevant features, factors and causes of the anomalous behavior. |
2.5 | Actionability | The method should be able to provide useful and actionable insights for the data owners, analysts and users, and suggest possible solutions or interventions for the detected anomalies. |
AI Data Protection
Embeds privacy, rights and governance mechanisms into data management via encryption, access controls, and consent procedures.
Control | Principle | Description |
---|---|---|
3.1 | Data Minimization | The method should collect and process only the minimum amount of data that is necessary and relevant for the AI purpose, and delete or anonymize the data when it is no longer needed. |
3.2 | Purpose Limitation | The method should ensure that the data is used only for the specific and legitimate AI purpose that was consented by the data subjects, and not for any other incompatible or unlawful purposes. |
3.3 | Consent and Control | The method should obtain the informed and explicit consent of the data subjects for the collection and processing of their data for AI, and provide them with the ability to access, rectify, erase, restrict or object to the use of their data. |
3.4 | Security and Integrity | The method should protect the data from unauthorized or accidental access, disclosure, alteration, loss or destruction, using appropriate technical and organizational measures, such as encryption, authentication and backup. |
3.5 | Accountability and Auditability | The method should document and demonstrate the compliance with the data protection laws and regulations, and enable the verification and evaluation of the data protection practices and outcomes by internal or external auditors. |
Model Operations
Oversees responsible model development, testing, deployment, monitoring and updates over the machine learning lifecycle.
Control | Principle | Description |
---|---|---|
4.1 | Automation | The method should automate the deployment, testing, validation, monitoring and updating of AI models, using tools and platforms that support continuous integration and delivery (CI/CD) of AI applications. |
4.2 | Scalability | The method should be able to handle the increasing volume, variety and velocity of data and models, and provide flexible and elastic solutions that can scale up or down according to the demand and resources. |
4.3 | Reproducibility | The method should be able to track and document the data sources, transformations, features, parameters, versions and results of AI models, and enable the replication and verification of the model outcomes. |
4.4 | Collaboration | The method should facilitate the communication and coordination among the data engineers, data scientists, AI developers, business analysts and other stakeholders involved in the AI lifecycle, and foster a culture of feedback and learning. |
4.5 | Governance | The method should ensure the compliance with the technical, ethical, legal and regulatory standards and policies for AI, and provide mechanisms for oversight, accountability and auditability of the AI models and applications. |
Adversarial Attack Resistance
Verifies model robustness and detects vulnerabilities to various failure modes or malicious perturbations to drive safety and reliability.
Control | Principle | Description |
---|---|---|
5.1 | Detection | The method should be able to detect and identify the presence and type of adversarial attacks, using techniques such as anomaly detection, signature analysis and adversarial training. |
5.2 | Defense | The method should be able to defend and protect the data and AI models from adversarial attacks, using techniques such as input preprocessing, gradient masking and model regularization. |
5.3 | Recovery | The method should be able to recover and restore the data and AI models to their original state, using techniques such as backup, retraining and self-healing. |
5.4 | Diversity | The method should be able to increase the diversity and complexity of the data and AI models, using techniques such as data augmentation, ensemble learning and randomization. |
5.5 | Adaptability | The method should be able to adapt and evolve the data and AI models to cope with new and unknown adversarial attacks, using techniques such as online learning, reinforcement learning and meta-learning. |
Sustainable Technology Platform Engineering
Seeks to minimize long-term societal risks by aligning systems to human values and considering environmental impact.
Control | Principle | Description |
---|---|---|
6.1 | Carbon Efficiency | The method should measure and reduce the carbon emissions associated with the data and analytics platforms, using techniques such as carbon accounting, carbon offsetting and carbon pricing. |
6.2 | Energy Efficiency | The method should improve the energy efficiency and utilization of the data and analytics platforms, using techniques such as energy-aware scheduling, load balancing and power management. |
6.3 | Carbon Awareness | The method should incorporate the carbon intensity and availability of the energy sources into the data and analytics platforms, using techniques such as carbon-aware routing, carbon-aware scaling and carbon-aware optimization. |
6.4 | Hardware Efficiency | The method should leverage the hardware capabilities and features of the data and analytics platforms, using techniques such as hardware acceleration, hardware virtualization and hardware disaggregation. |
6.5 | Measurement | The method should monitor and report the environmental and performance metrics of the data and analytics platforms, using techniques such as telemetry, dashboarding and benchmarking. |
6.6 | Climate Commitments | The method should align the data and analytics platforms with the climate goals and policies of the organization, using techniques such as climate action planning, climate risk assessment and climate scenario analysis. |
Disclaimer
The views expressed on this site are personal opinions only and have no affiliation. See full disclaimer, terms & conditions, and privacy policy. No obligations assumed.